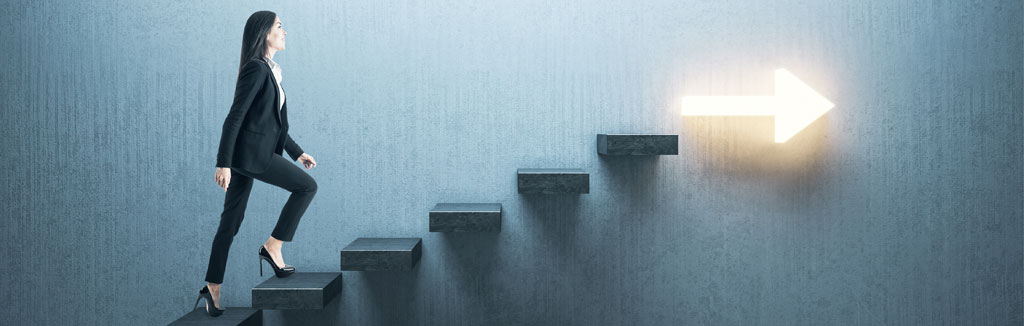
7 Steps to Building a Successful Customer Behavior Analytics Model
Having a comprehensive customer behavior analytics model is vital to ecommerce companies. Studies show that companies that use customer behavior information to their advantage outperform competitors by 85 percent in sales growth. Additionally, estimates show those who use behavior analytics beating competitors by an extra 25 percent in gross margins. The current influx of data available to measure customer behavior patterns is making it easier for ecommerce businesses to use analytics in the following ways:
- Support core sales and marketing goals
- Increase customer satisfaction rates
- Improve product/service criteria
- Optimize marketing channels
- Make insightful improvements to overall sales strategies
- Increase customer loyalty
Developing a customer behavior analytics model is vital to retail success for a variety of reasons, and ecommerce companies shouldn’t be without one. Read on to discover seven key ways to build the right customer behavior analytics model for your ecommerce business.
1. Use Detailed Data
As previously mentioned, there is an influx of big data in ecommerce businesses that help monitor customer behavior. Present analytics platforms can delve even deeper into consumer patterns, and tracking tools have become far more accurate. Estimates for the 2020 fiscal year suggest that ecommerce sales will pass over 4 trillion, and companies with a pulse on consumer behavior will take a larger chunk of those sales. The following forms of data can help retailers build a broad customer behavior analytics model:
- Order tracking: includes buying patterns, most purchased products, and reordering habits
- Consumer engagement: helps gauge what features, products, and information matters most to consumers
- Changes in order patterns: helps businesses anticipate possible upcoming ordering changes that an online consumer may go through
- Conversion rates: how many consumers convert from visitation to purchasing from a brand
- Retention rates: how many consumers remain with a brand long-term
- User feedback: customer satisfaction ratings, reviews, comments, and likes
The ultimate goal of using customer behavior analytics in retail is to get actionable insight into what consumers are looking for. Criteria for that can vary by company, which means the data needed can also vary; the list above gives forms of data that are useful to every ecommerce business. This is just a short list of ideas, and there are many forms of data that help ecommerce businesses build relevant models for customer behavior analytics. When determining what data to add, keep all major business specific KPIs in mind to get the best possible analysis of consumer behavior.
2. Throw Out the RFM Model
Use a life-time value (LTV) model instead of a recency, frequency, and monetary (RFM) model. Ecommerce businesses in particular benefit from using LTV over RFM. Digital tracking and an increase in the accuracy of analytics platforms has made RFM models a poor choice for customer behavior analytics. The reason they aren’t a good fit is because the majority of the key indicators for that model are based on immediate sales. They also primarily focus on those who spend the most money, and they lack any tangible insight into retaining new customers long-term. LTV models can provide actionable insight to take for individual consumers, target their long-term value to the ecommerce business, and select appropriate marketing for them.
3. Stop Making Assumptions Based on Age, Demographics, or Finances
There are 35-year-old people who watch cartoons, and believe it or not, that’s an excellent point of reference for this segment. Customer behavior isn’t measured by simply lumping age groups, demographics, or income levels into tidy piles and assuming what they will or will not respond to. Making assumptions about customer behavior patterns is not conducive to developing a successful or accurate customer behavior analytics model. The goal is to start thinking of customers as individuals, not groups. A 70-year-old can buy hiking gear and a 20-year-old may purchase knitting needles. To get the best possible results, find out what individual consumers want by basing analytics on real data and not assumptions. In short, don’t stereotype.
4. Use Predictive Analysis
Predictive analysis is an excellent way to draw in potential customers, keep existing customers, and anticipate future needs in order to suggest relevant products. Predictive analytics drive the following ecommerce metrics:
- Customer acquisition: helps by tracking the consumer’s journey from the initial site visit to checkout to personalize their experience
- Customer retention: helps by anticipating problem areas to repair based on data such as customer feedback or drop rates
- Customer growth: helps retailers create calls to action based on ordering patterns during specific periods
Personalized advertising, suggestions, and promotions can all be tailored to customers using data obtained from predictive analysis. Using predictive analytics to understand the behavior of customers is another way to use big data in ecommerce to make changes that improve retention.
5. Know Your Goal and Create Steps to Reach It
Building a comprehensive customer behavior analytics model means knowing what goals your ecommerce company wants to achieve. There are many steps to building customer behavior analytics that companies can take advantage of to reach goals, including :
- Set analytics goals and KPI’s, and track them
- Determine critical paths, then break them up to get the most data
- Set user properties to receive data on customers using your site
- Continually monitor and adapt analytics models based on consumer practices and new data
- Measure success of new products to determine their impact on sales
- Use funnel analysis methods
Having specific goals in mind while developing customer behavior analytics helps ecommerce businesses make marketing and advertising decisions and changes based on reliable data.
6. Incorporate Funnel Analysis
Funnel analysis is particularly useful for determining abandonment rates through each stage of the checkout process. They also help establish the set of steps consumers must go through to reach any specific outcome on a website. Funnel analysis helps ecommerce organizations visualize data by showing drop off points. For this reason, these types of analytics are ideal for verifying drop rates, tracking site abandonment, and showing weaknesses that exist in each stage of the process. Funneling is also an excellent tool for ascertaining why conversions were unsuccessful.
7. Search Out Customer Access Points
Are you getting the most customer engagement from external or internal links? Are they accessing the site through social media posts or click ads? It’s important to pinpoint consumer access points for your brand and track behavior across all points. This type of behavioral analysis helps businesses target areas that produce the most clicks and conversions. That way, retailers can position ads appropriately to maximize their potential draw. Being able to allocate resources properly is one of the key benefits of analyzing consumer behaviors. For customer behavior analytics models to be successful, they have to include data from all areas of the business that consumers have access to. Access areas to include during the process of developing a customer behavior analytics model include:
- Primary website: main ecommerce site
- Apps: downloads, uninstalls, in-app purchases, and feedback
- Social media: Facebook®, Twitter®, Instagram®, etc.
- Click ads: any advertisement that provides a direct link to primary site
- External links: links on other sites that direct users to primary site or other company resource
Metrics measurements should span across any location that gives users access to your brand, or they are not truly accurate. Using data from websites alone can give a lopsided view of customer behavior, because not everyone will access your product through the main site. It all circles back to the importance of analyzing customer behavior on an individual level.
The insight gained into the personal habits of customers is crucial to recommendation searches, email campaigns, and product suggestions. Do you have any insights into creating a successful customer behavior analytics model? Comment so readers can add them to the information they gained from this post. If you still need guidance on how meet your ecommerce consumer goals, contact eZdia where our professionals will be more than happy to help you succeed.
Author: Kristin Ann Hassel
eCommerce Content Optimization
Want to learn more about how our eCommerce content services can help you to outrank your competitors?